Transforming Pricing Strategies in Manufacturing with AI, Machine Learning, and Big Data
AI, machine learning, and big data are reshaping manufacturing pricing strategies, offering new ways to enhance precision, adapt to market changes, and optimize profit margins. This article explores the transformative power of these technologies and the best practices for overcoming challenges in data quality, transparency, and compliance.
In today’s manufacturing sector, pricing strategies are critical for balancing competitiveness and profitability amid increasing market complexity. Traditional models—often static and cost-based—struggle to adapt to rapid changes in demand, input costs, and customer expectations. Enter AI, machine learning (ML), and big data: these technologies are enabling a shift to dynamic, data-driven pricing.
However, leveraging AI-driven pricing strategies introduces new challenges, from the need for transparent customer interactions to navigating a regulatory landscape that’s evolving almost as quickly as the technology itself. How can manufacturers harness these tools while addressing such pressing concerns?
The Transformative Role of AI and Machine Learning in Pricing
Enhanced Pricing Accuracy through Predictive Analytics
AI and ML bring new levels of precision to pricing by analyzing extensive datasets—including historical sales, competitor trends, and current market shifts—to detect patterns and inform proactive pricing decisions. Predictive analytics enables manufacturers to anticipate trends, like seasonal demand fluctuations or spikes in input costs, with unprecedented accuracy. But is this precision easy to achieve?
Consider GE’s approach. Within its industrial equipment division, GE integrates ML models to adjust pricing based on demand forecasts and raw material cost variations. By using predictive analytics, GE has enhanced its market responsiveness and safeguarded profit margins during volatile periods. However, implementing this predictive capability across divisions has required substantial infrastructure investment and continuous data refinement.
With the increasing regulatory focus on AI applications, companies like GE face the added responsibility of transparency. As regulatory standards evolve, questions about fairness in AI-driven pricing will likely increase, urging companies to maintain clear, consumer-centered pricing practices to avoid potential backlash.
"Big data allows manufacturers to create pricing strategies that reflect a deep understanding of customer behavior, competitive dynamics, and broader economic indicators..."
Dynamic Pricing Models for Real-Time Adaptability
Dynamic pricing, once primarily seen in e-commerce, is making significant inroads into manufacturing as AI enables real-time adjustments. AI-powered systems can adapt prices instantly, responding to variables such as inventory levels, lead times, and raw material costs. This flexibility allows manufacturers to capitalize on high-demand periods and protect margins during downturns. But can this approach always align with customer expectations?
In the automotive industry, Ford’s adoption of dynamic pricing illustrates both its benefits and the considerations it demands. Ford adjusts prices in response to supply chain disruptions, which has strengthened its margin stability and helped the company maintain competitive positioning during shortages.
Initially, however, this shift to dynamic pricing led to customer concerns about perceived volatility. To address this, Ford launched a proactive communication strategy to explain the benefits of adaptable pricing and the factors driving price changes. For B2B manufacturers, achieving a balance between responsiveness and consistency will be essential as they navigate customer loyalty in an era of fluctuating prices.
Market Insights from Big Data
Big data allows manufacturers to create pricing strategies that reflect a deep understanding of customer behavior, competitive dynamics, and broader economic indicators. By analyzing this wealth of information, companies can develop region-specific pricing that aligns with customer needs and market conditions. However, with such extensive data comes the challenge of maintaining data integrity.
Siemens exemplifies the effective use of big data for market-specific pricing. By tailoring prices to regional demand, Siemens has improved its margin stability while enhancing customer retention in price-sensitive markets.
Yet, maintaining data accuracy requires continuous investment, particularly as regulatory demands around data privacy tighten globally. For Siemens, compliance with data privacy laws has become as critical as data quality itself, as failure to adapt could compromise customer trust and brand reputation. As big data becomes integral to pricing, manufacturers will need both robust compliance measures and adaptive data strategies to stay competitive.
Overcoming Challenges in Technology Integration
Data Management and Quality Concerns
AI, ML, and big data rely on high-quality data to function effectively. Poor data quality can lead to skewed AI predictions and misinformed pricing decisions that may harm profitability and customer relationships. For many manufacturers, establishing a comprehensive data governance framework is not only advisable but essential. Still, data management remains a formidable task.
Bosch has made significant strides by centralizing data across divisions to minimize silos and enhance consistency in decision-making. This centralized approach has improved Bosch’s pricing accuracy, contributing directly to greater profit stability.
However, maintaining data quality on a global scale is an ongoing challenge that requires substantial resources. In today’s data-centric world, Bosch’s approach underscores a vital reality: data management is no longer merely operational; it is a strategic priority that supports both compliance and competitive advantage.
Integration and Cost Implications
Deploying AI-driven pricing solutions involves substantial initial investment, from infrastructure upgrades to hiring skilled talent.
Not all manufacturers have the resources for a full-scale transformation. To manage costs, some companies, like Honeywell, have taken a phased approach, piloting AI in specific divisions and assessing results before expanding further.
Cloud-based AI solutions provide another viable option, enabling companies to enhance AI capabilities without the need for extensive hardware investment. However, cloud adoption also raises data security and regulatory compliance concerns. As data privacy laws become more stringent, manufacturers must implement robust data protection measures to ensure compliance.
"Manufacturers implementing AI-powered pricing are already seeing improved profitability and customer retention..."
For Honeywell, and others relying on cloud solutions, balancing cost-effectiveness with stringent compliance is no longer optional. How can companies fully embrace cloud-based pricing without compromising security?
In an era of rapid regulatory change, adaptable compliance frameworks are crucial.
Practical Examples of Technology-Enhanced Pricing
Manufacturers implementing AI-powered pricing are already seeing improved profitability and customer retention. For instance, in electronics, companies use AI-driven demand forecasting to adjust prices during peak periods, allowing them to capture higher margins while remaining competitive in price-sensitive segments. By aligning profit goals with customer preferences, these companies demonstrate how AI can enhance both financial performance and customer satisfaction.
Similarly, Caterpillar’s approach in the heavy equipment sector exemplifies the tangible impact of machine learning on margin stability. By adjusting prices in response to fluctuations in raw material costs, Caterpillar has preserved profit margins during volatile periods.
Yet, this system requires ongoing model updates to retain accuracy—a reality that many new AI adopters overlook. For Caterpillar, AI has proven invaluable in driving cost efficiency and resilience, underscoring that machine learning, when properly maintained, can be a formidable asset in a turbulent market.
Looking Ahead: The Future of Technology in Pricing Strategy
As AI, ML, and big data reshape manufacturing, several emerging trends will define the future of pricing strategies:
Increased Automation:
Advanced AI models promise to automate more pricing functions, reducing the need for manual oversight and allowing manufacturers to respond faster to market shifts. But can pricing really be fully automated? Even as automation capabilities improve, maintaining oversight will remain crucial to align AI decisions with broader business objectives and ethical considerations, particularly as regulators scrutinize algorithmic influence on pricing practices.
Personalized Pricing Models:
In B2B sectors, AI’s capacity for analyzing client-specific data is facilitating more personalized pricing, especially for high-value customers. By tailoring prices based on order volume or historical needs, manufacturers can drive loyalty while maximizing profitability. Yet, as personalized pricing becomes more common, companies will need to prioritize transparency. With customers and regulators increasingly concerned about fairness, ethical considerations are no longer a mere afterthought—they’re essential for long-term success.
Sustainable Pricing Strategies:
Sustainability is rapidly becoming a key factor in competitive pricing. AI models are beginning to incorporate environmental factors, such as carbon footprint, into pricing structures, aligning with consumer demand for eco-friendly products. However, implementing sustainable pricing is challenging without standardized metrics for environmental impact. Manufacturers aiming to lead in sustainability will need to adopt adaptable pricing models that can evolve alongside changing industry standards.
"Embracing these technologies isn’t just an advantage—it’s a strategic imperative in today’s rapidly evolving marketplace..."
Best Practices for Adapting Technology-Driven Pricing Models
To fully capitalize on AI, ML, and big data in pricing, manufacturers should consider the following best practices:
Data Governance and Quality Assurance:
High-quality data is crucial for reliable AI insights. Regular audits and strict data protocols are essential to avoid inaccuracies that could distort pricing decisions.
Flexible System Architecture:
Cloud-based solutions provide scalability, enabling manufacturers to expand AI capabilities as market conditions evolve without the need for extensive hardware investments. This flexibility is invaluable in a volatile market landscape.
Cross-Department Collaboration:
Effective pricing strategies benefit from insights across departments—production, finance, sales, and customer service. By fostering collaboration, manufacturers can ensure that pricing decisions reflect comprehensive operational needs and customer expectations, delivering a balanced and competitive approach.
In conclusion, AI, ML, and big data are set to redefine pricing in manufacturing, enabling a new level of precision, responsiveness, and market insight. While challenges in data quality, regulatory compliance, and customer trust persist, manufacturers who proactively address these issues stand to gain a considerable competitive edge.
Embracing these technologies isn’t just an advantage—it’s a strategic imperative in today’s rapidly evolving marketplace.
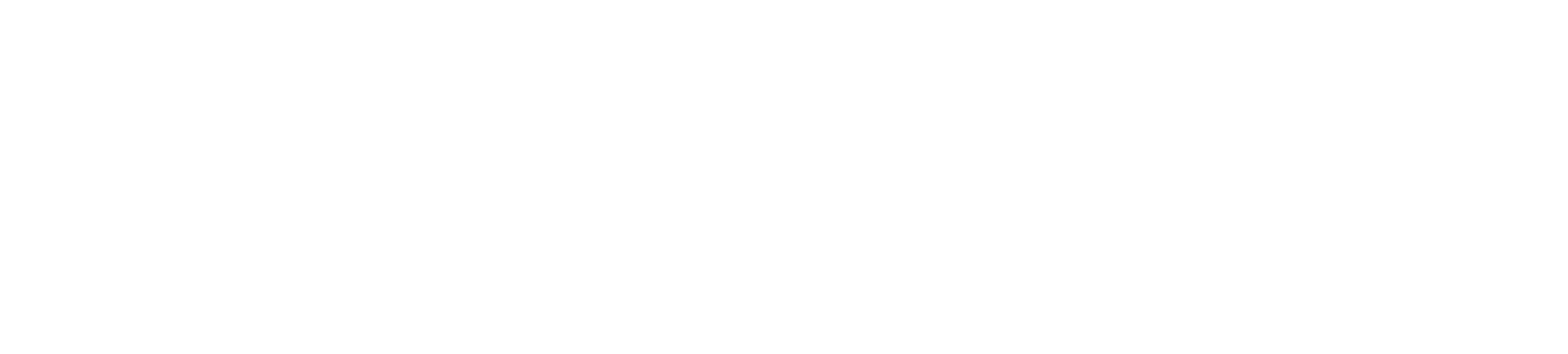