Proactive Maintenance: Harnessing Predictive Analytics to Stay Ahead in Field Service
In the high-stakes world of field service, maintenance is no longer about scrambling to fix broken equipment. It’s about seeing issues coming—knowing what might fail and when so teams can step in early, avoid costly downtime, and keep customers satisfied…
Predictive analytics is at the core of this shift, transforming maintenance from a reactive “fix it when it breaks” model to a proactive “catch it before it fails” strategy. But how exactly does this work, and what benefits does it bring? Let’s dive into how industry experts see this trend reshaping field service.
As Johann Diaz, Founder of Service Revolution Academy, puts it, “Predictive analytics is changing the game in field service by enabling what’s known as predictive maintenance. Instead of waiting for equipment to break down or doing routine check-ups regardless of need, both of which cause significant increased costs, predictive maintenance lets teams see potential issues before they happen, thanks to real-time data from sensors.”
Here, Diaz’s point is simple but profound: predictive maintenance isn’t just about keeping machines running—it’s about fixing issues at just the right moment, when needed, and without the costs or surprises of unexpected downtime. “This means repairs happen only when they’re actually needed, saving time, cutting costs, and keeping customers happy by avoiding surprise downtimes,” he adds.
With IoT sensors and AI-powered analytics, field service teams can spot minor performance shifts early on. This means they’re not just responding to problems but actively preventing them.
As Diaz notes, “With tools like IoT sensors and AI, field service teams can catch minor changes in performance early, scheduling fixes before they escalate into full-blown breakdowns. This proactive approach reduces downtime, keeps operations smooth, and allows for better inventory planning since you can better forecast, with greater accuracy, which parts might be needed soon.” The takeaway? Predictive maintenance is more than just a clever tool; it’s a foundational shift toward seamless, customer-focused service.
From Reactive to Proactive: The Competitive Edge of Timing
For years, maintenance has typically been reactive, with teams rushing to fix issues as they happen, or it’s been rigidly scheduled, which often leads to unnecessary costs. Predictive maintenance offers an alternative approach, enabling field service companies to stay one step ahead, resolving issues before they interrupt service. And in a competitive environment, that edge is crucial.
Victoria Poku, Senior Solution Consultant at ServiceNow, sees predictive analytics as key to helping field service companies intervene “much earlier in the lifecycle—and meet their Service Level Agreements far more consistently.”
This ability to prevent disruptions is not just convenient; it’s essential in high-stakes industries where reliability is a must. But predictive tools offer more than early detection; they help identify patterns in recurring issues, providing a big-picture view of underlying problems.
“Change management is crucial, as teams may be resistant to relying on analytics; regular training, transparent communication, and highlighting early successes help build trust and adoption...” - Frank Klomp, Optimize My Day
“We can now automatically group together cases that have a common underlying cause into ‘work order trend topics.’ These can speed up resolution and reduce backlogs. For example, managers can act quickly to resolve clusters of cases, rather than tackling them individually. And agents feel much more empowered, as they can proactively resolve issues by analysing other work orders in the topic,” Poku explains.
It’s a powerful shift—from a reactive scramble to a proactive assurance that systems will keep running smoothly.
Navigating the Challenges of Implementing Predictive Analytics
Adopting predictive maintenance, however, isn’t without its roadblocks. For predictive models to be accurate, they need high-quality, consistent data, which can be difficult to obtain, especially with legacy equipment. So, how can field service teams overcome these data challenges?
Frank Klomp, Managing Director of Optimize My Day, stresses the importance of reliable data. “Data is key. Many service companies struggle with collecting consistent, high-quality data,” he notes. To address this, many are turning to advanced data warehousing tools like OMD’s Powerhouse, which unifies and processes data for predictive insights.
“OMD’s Powerhouse is such a warehouse combining data ingestion with intelligent forecast-models based on AI to improve the predictive capabilities of service organizations,” Klomp explains. But data quality isn’t the only issue—many companies are also working with older equipment that lacks IoT compatibility. As Klomp points out, “The installed base, older systems in particular, may lack the appropriate sensors and IoT technologies to send live data directly to the data collectors. They must be modernized or replaced in order to improve the forecasting capacity.”
There’s also the human side to consider. Shifting teams from traditional maintenance to predictive models means rethinking processes and helping teams trust data-driven insights over hands-on experience. “Change management is crucial, as teams may be resistant to relying on analytics; regular training, transparent communication, and highlighting early successes help build trust and adoption,” Klomp says. And he’s right—it’s not just about bringing in new tools but creating a culture that values predictive insights as a core part of the job.
Measuring ROI: Tracking Predictive Maintenance’s True Impact
For companies weighing an investment in predictive maintenance, proving ROI is essential. Predictive analytics can yield significant benefits, from fewer emergency repairs to improved First-Time Fix Rates and extended equipment life. But how can companies quantify these gains?
Poku advises that ROI should be approached with clear metrics and tools that make analysis simple. “There are two key parts to this in my view. The first is deciding what data points you need to measure. And the second is how you bring all those together into one place and turn them into usable insights,” she says. ServiceNow has created preconfigured dashboards that offer a consolidated view of key performance metrics in real time.
“Typically, companies will want to track the status of work orders, time to resolution, agent utilisation, and so on. But this data is only really useful if you can analyse it effectively,” Poku explains. A comprehensive, accessible dashboard allows managers to see exactly where predictive maintenance is driving results. “In short, if the right data is presented in a convenient, usable format, customers can better track their cost allocations and get an accurate view of the ROI of their predictive maintenance tools.” Ultimately, predictive maintenance’s value isn’t abstract—it’s measurable and actionable.
The Future of Predictive Analytics: AI, Edge Computing, and Digital Twins
Looking ahead, the potential for predictive maintenance continues to expand, especially with the rise of AI, edge computing, and digital twins. What could these new technologies mean for the future of field service?
Johann Diaz sees edge computing as a particularly exciting development. “One of the most exciting future developments in predictive analytics is the increased integration of AI-driven edge computing with IoT sensors. Edge computing allows data processing to happen at the device level, enabling near-instantaneous analysis without relying on centralized servers.
"By combining predictive analytics with digital twins, field service teams can simulate various maintenance scenarios, test solutions virtually, and accurately predict failure points before they occur..." Johann Diaz, Service Revolution Academy
This will allow field service teams to make faster, real-time maintenance decisions. For industries where equipment uptime is critical, edge computing could significantly reduce latency in predictive maintenance, enhancing the timeliness of interventions.”
Self-learning AI models are also set to play a key role. These tools don’t just follow static patterns—they adapt continuously based on real-world data. “Unlike traditional models that need regular human oversight for tuning, self-learning models can adapt to new patterns, increasing the accuracy of predictions over time without requiring constant reprogramming. This will enable predictive maintenance systems to become more accurate with minimal human intervention, paving the way for more autonomous field service operations,” Diaz explains.
Another game-changer is the use of digital twins—virtual replicas that mimic the real-time state and performance of physical equipment.
“The growing application of digital twins in predictive maintenance is incredibly exciting. A digital twin is a virtual replica of physical equipment that mirrors its real-time state and performance. By combining predictive analytics with digital twins, field service teams can simulate various maintenance scenarios, test solutions virtually, and accurately predict failure points before they occur,” Diaz says.
It’s a smart approach that could drastically improve planning, allowing companies to virtually test solutions before implementing them on-site.
Klomp, too, sees potential in integrating external data sources, noting, “In the future, more and more external circumstances, such as weather models, economic developments, political decisions and supply chain constraints will influence predictive maintenance. It is particularly important then to be able to intelligently combine and evaluate all available data sources.” Incorporating broader external data could make predictive models even more accurate, enabling teams to respond to a full spectrum of influences.
Data Accuracy: The Backbone of Predictive Success
The success of predictive analytics hinges on one thing above all else: data accuracy. Without it, even the best technology can’t provide reliable insights. “Regular data validation, maintaining data governance standards, and cleaning data frequently to avoid bias and errors in predictive models by the help of automated validation tools,” says Klomp, are essential for effective predictive maintenance.
High-quality IoT sensors, consistent validation protocols, and model retraining ensure predictive tools stay aligned with real-world conditions. Self-monitoring algorithms and regular data cross-checks make predictive analytics not only reliable but dynamic, adjusting to changes in equipment behavior over time.
Conclusion: The Future of Field Service is Predictive
Predictive analytics is changing field service in a profound way, turning maintenance from a reactive responsibility into a proactive strategic advantage. For field service companies, predictive maintenance isn’t just about keeping machines running—it’s about creating reliable, data-driven systems that support uptime, efficiency, and customer trust.
With continuous advances in AI and IoT, predictive maintenance will only become more integral, helping companies stay ahead in an increasingly competitive landscape. The future of field service is clear: it’s proactive, predictive, and here to stay.
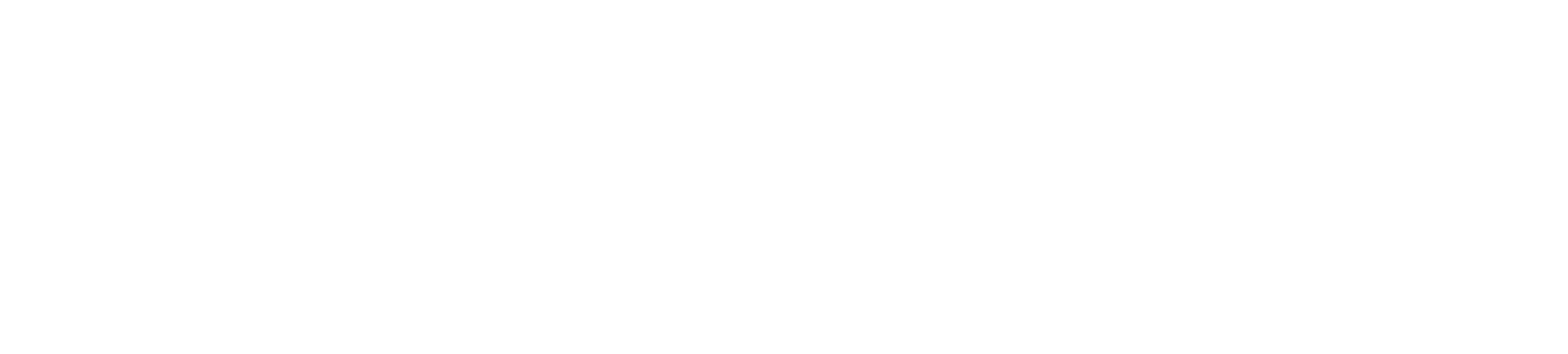